About the NVIDIA H200
Amid the release of NVIDIA’s next-generation GPU for AI, the NVIDIA Blackwell architecture, they have refreshed and revamped their NVIDIA Hopper lineup with an all-new deployment option for increased compute power. The NVIDIA H100 was undoubtedly the pinnacle of AI performance powering LLMs and generative AI. With AI becoming deeply engrained in changing the landscape of every industry, the need for a strong AI accelerator like the NVIDIA H200 featuring increased memory from 80GB HBM3 to 141GB HBM3e as well as increased memory bandwidth up to 4.8TB/s
But that begs the question; “Does my organization need a system with NVIDIA H200?”
NVIDIA H200 Specifications
NVIDIA H200 comes in 2 options — HGX and PCIe.
- NVIDIA HGX H200: The NVIDIA HGX H200 is the data center SXM5 variant featuring 4 or 8 interconnected GPUs via NVLink for increased GPU-to-GPU communication essential for workloads that require multiple GPUs to work together.
- NVIDIA H200 NVL: The NVIDIA H200 NVL is a more mainstream option in the PCIe form factor that uses NVLink bridges for interconnecting GPUs. However, the H200 NVL is likely more suitable for workloads that parallelize the workload across multiple GPUs.
The main difference between HGX and H200 NVL is scalability. HGX H200 can be further scaled with multiple HGX systems for an even more interconnected GPU deployment. The fast NVLink interconnect and NVLink Switch system enables these GPUs to communicate at lightning-fast 4.8TB/s speeds.
H200 SXM | H200 NVL (PCIe) | |
Deployment | NVIDIA HGX - 4 Way or 8 Way with NVLink | 2 Way or 4 Way NVLink Bridge |
Form Factor | SXM5 | PCIe 5.0 |
GPU Memory | 141GB HBM3e | 141GB HBM3e |
Memory Bandwidth | 4.8TB/s | 4.8TB/s |
FP64 | 34 TFLOPS | 30 TFLOPS |
FP64 Tensor Core | 67 TFLOPS | 60 TFLOPS |
FP32 | 67 TFLOPS | 60 TFLOPS |
TF32 Tensor Core | 989 TFLOPS | 835 TFLOPS |
FP16 Tensor Core | 1979 TFLOPS | 1671 TFLOPS |
FP8 Tensor Core | 3958 TFLOPS | 3341 TFLOPS |
TDP | 700W Configurable | 400-600W Configurable |
NVIDIA H200 Use Cases
NVIDIA H200 in both HGX and NVL options is suitable for training AI, real-time data analytics, engineering simulation, and HPC.
- Training Foundational Models & Complex AI
- Real-Time Data Analytics
- Weather Modeling, Prediction Algorithms
- Engineering Simulation
- FEA, CFD, Molecular Dynamics
We will go over what kinds of deployments are best suited to fully utilize a deployment equipped with NVIDIA H200 and highlight which H200 (HGX vs PCIe) would be best.
Training Foundational Models & Complex AI
NVIDIA’s endeavors in developing the Tensor Core GPUs like the A100, H100, and now H200 have all been hyper-focused on accelerating AI training performance. As AI models become larger and larger, the need for interconnected GPUs led to the further development of NVLink technology.
Training foundational AI models for LLMs and Generative AI necessitates huge amounts of data and thus a huge GPU memory capacity to reduce callbacks to solid-state storage. If an AI model can perform its calculations on the neural network straight off GPU memory, you can limit data fetch bottlenecking.
Furthermore, training novel AI models for your workloads like fraud detection, recommendation systems, and other real-time data analysis can also benefit from the added performance of NVIDIA H200. But in this case, storing the entire model in GPU memory is not quite as essential. The H200 NVL PCIe version will be sufficient.
However, this mainly applies to only the training and powering of these foundational AI models. Once the model is trained, inferencing is significantly less compute-intensive. But that doesn’t mean H200 is not needed anymore. Companies that host their AI as an API call will require a multi-instance where each prompt can be tackled in parallel.
Real-Time Data Analytics and Modeling
Weather Modeling, Seismic Processing, Data Analytics and Data Science. All these workloads require high-performance memory bandwidth and unified GPU architecture for quick GPU intercommunication.
Real-time data workloads can take advantage of NVIDIA H200 due to the high GPU memory bandwidth providing faster data access and reduction in GPU-to-GPU bottlenecks. NVIDIA H200’s HBM3 memory is capable of over 4.8TB/s of memory bandwidth, more than any other component, just make sure other components like data ingest/storage can handle the speed.
However, there is a precaution that an NVIDIA H200 deployment can be overkill. If the model is not large, there will be idle resources, leading to a lower return on investment. Applications like fraud detection machine learning algorithms can utilize a pair of NVIDIA H200 NVLs in a smaller form factor server or utilize lower-tiered GPUs like NVIDIA RTX 5000 Ada that will perform admirably.
Engineering Simulation
Engineering simulations are critical in numerous fields, from aerospace to automotive and civil engineering. These simulations require substantial computational power to model complex systems accurately. As the systems gain more complexity, the larger the memory bus per GPU is required to fit and run simulations on the model.
Deployments equipped with NVIDIA H200 GPUs offer significant benefits in accelerating these simulations due to its over-the-top 141GB of HBM3 memory. There is no GPU on the market that comes close to the total memory per card, not to mention the capability of running multiple NVIDIA H200.
Suppose your simulation cannot be split between multiple interconnected GPUs (which is often the case). In that case, models must be loaded on every GPU in the deployment in which various calculations can be performed in parallel (if applicable) like CFD (computational fluid dynamics) and particle dynamics. Deformation and FEA-type simulations are often sequential, but some have GPU acceleration capabilities.
The biggest upside to deploying NVIDIA H200 is its native double-precision FP64 capability. Some simulations require the utmost precision, even by a fraction of a decimal. If your workload requires floating point precision flexibility, the only GPUs in NVIDIA’s lineup that have FP64 native solvers is the H200 NVL (PCIe form factor), HGX H200 (SXM form factor), and A800 40GB Active (PCIe form factor). Housing big models mean H200 NVL is the best choice.
When to Deploy NVIDIA HGX vs H200 NVL?
AI Training | AI Inferencing | Simulation | |
---|---|---|---|
8 or 10 GPU Server | Large Foundational Models | Not Needed | Large Simulation (over 20 million cells) |
4 GPU Server or Rackmount | Complex AI Models | Large AI Models | Medium to Large Simulation (10-20 million cells) |
2 or 4 GPU Server or Rackmount | Small-Scale Data Analytics | Small to Medium size AI Models | Smaller Simulations (Less than 15 million cells) |
Evaluate your workload's size and determine if the GPU bandwidth, memory, and interconnect difference will drastically improve performance. The NVIDIA H200 is all about accelerating what’s big, like LLMs, large simulations, complex models and prediction algorithms, and more.
If you need help evaluating your computing needs, do not hesitate and contact SabrePC today. Our highly experienced engineers custom-tailor and build HPC deployments to meet your unique computing demands. Click the link below to view our NVIDIA H200 and H100 platforms to configure.
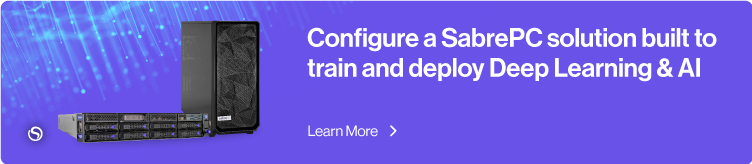