Introduction
Artificial intelligence is rapidly advancing, with distinct branches emerging that offer unique capabilities. While many are familiar with AI's capacity to generate content, a newer paradigm is gaining prominence: Agentic AI.
Agentic AI involves a more complex system that includes planning, decision-making, and interaction with its environment to accomplish defined objectives.
This blog will explore the fundamental differences between these two forms of AI, detailing their respective capabilities, applications, and potential implications and comprehensive understanding of their roles in the evolving AI landscape and their anticipated impact on various industries.
What is Agentic AI?
Agentic AI refers to artificial intelligence systems designed to operate autonomously with a specific goal or task in mind. These systems mimic human-like decision-making capabilities, enabling them to navigate dynamic environments and adapt to changing circumstances. At its core, Agentic AI leverages perception, reasoning, and action to achieve its objectives without continuous human intervention.
Key Characteristics of Agentic AI:
- Goal-Oriented Behavior: Agentic AI systems are programmed with explicit objectives, such as navigating a route or optimizing a process.
- Autonomous Operation: They make decisions and take actions independently based on environmental feedback.
- Dynamic Adaptability: These systems adjust to changes in their environment to remain effective.
Agentic AI finds applications in a variety of fields, such as:
- Autonomous Vehicles: Self-driving cars use sensors and AI algorithms to perceive their surroundings, make decisions, and navigate safely.
- Industrial Automation: Robots in manufacturing settings autonomously execute tasks like assembly and quality control.
- Customer Support: AI systems assist customers by analyzing help desk comments or issues they have and suggesting potential remedies or routing to the appropriate resource.
By focusing on autonomy and adaptability, Agentic AI drives innovation in environments that require real-time decision-making and execution.
What is Generative AI?
Generative AI specializes in creating new content, such as text, images, music, and videos, by analyzing patterns and data. Unlike Agentic AI, it is not goal-driven in the traditional sense but is instead designed to mimic creativity and produce outputs based on prompts or training inputs. This type of AI utilizes advanced machine learning models, particularly deep learning frameworks, to generate realistic and coherent results.
Key Characteristics of Generative AI:
- Content Generation: It excels at creating original content by synthesizing information from its training data.
- Prompt Dependency: Generative AI relies on user-provided prompts or inputs to guide its output.
- Pattern Recognition: It identifies and replicates patterns from its training data to produce high-quality results.
Applications of Generative AI are widespread and growing rapidly, including:
- Creative Industries: Tools like ChatGPT generate written content, while DALL·E creates digital art.
- Prototyping and Design: AI models assist in ideating and visualizing products in fields such as marketing and product development.
- Data Augmentation: Generative AI enriches datasets for training machine learning models by creating synthetic examples.
Generative AI's ability to produce high-quality content has made it an indispensable tool for industries seeking to enhance productivity and creativity.
Agentic AIs can employ various types of generative AI to achieve their goals. They are often built on multiple models to help with reasoning and generation. For example, a customer service agent will utilize reasoning to comprehend and solve an issue and use generative AI to communicate that to the end user.
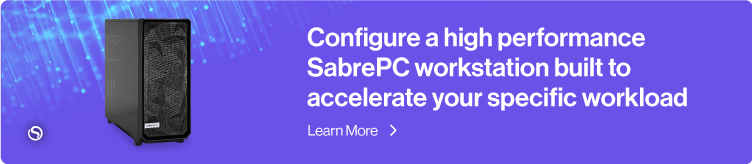
Comparing Agentic AI and Generative AI
While Agentic AI and Generative AI share the broader goal of leveraging artificial intelligence to improve efficiency and innovation, their core purposes and functionalities set them apart. Agentic AI is fundamentally task-driven, designed to autonomously solve real-world problems by perceiving, reasoning, and acting within dynamic environments. In contrast, Generative AI is creativity-driven, specializing in producing content based on learned patterns and user prompts.
Key Differences:
- Purpose and Goals:
- Agentic AI: Focused on achieving predefined objectives through decision-making and adaptability.
- Generative AI: Geared toward generating creative outputs that mimic human creativity.
- Core Capabilities:
- Agentic AI: Excels in real-time decision-making and autonomous execution.
- Generative AI: Excels in pattern recognition and content synthesis.
- Dependency:
- Agentic AI: Operates with minimal human intervention after deployment.
- Generative AI: Relies heavily on user inputs and prompts to function effectively.
Despite these differences, the two paradigms often complement each other in practical applications. For instance, Agentic AI systems could leverage Generative AI to create contextual data or content for specific tasks. Emerging hybrid models are integrating generative capabilities into autonomous systems, enabling more robust and flexible AI solutions that use a mixture of various models to perceive, calculate, and evaluate the situation, problem, or process.
This could go the other way too where a chatbot, which is often characterized as a generative AI, is actually an agentic AI. We don't know the underlying architecture behind our favorite AI chatbots, but they could be using an agentic AI type model powered by traditional prompt and output structure for highly specialized tasks that require a lot of reasoning such as code copilot, solving math problems, and more.
Conclusion
Agentic AI and Generative AI represent two transformative yet distinct paradigms within the AI ecosystem. While Agentic AI focuses on autonomous, goal-driven actions, Generative AI specializes in producing creative outputs based on learned patterns. Their differences highlight the diverse ways AI can contribute to innovation, from streamlining decision-making processes to augmenting human creativity. The convergence of these paradigms through hybrid models offers exciting possibilities, enabling systems that combine autonomy with creativity.
Planning on deploying your own Agentic AI or Generative AI model in your local organization? SabrePC excels in delivering custom configurable HPC workstations and servers perfect for running the inference of foundational AI models. Explore our Deep Learning Solutions for a free quote on a custom configuration or contact us today for more information!
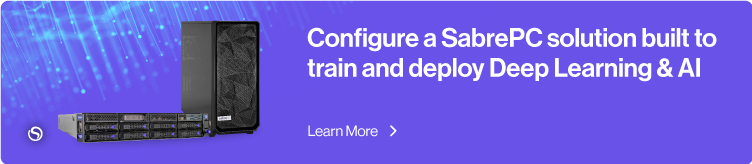