What Causes Large Language Models to Hallucinate
Large language models have revolutionized natural language processing by generating human-like text. Models like ChatGPT, DALLE-2, Google Bard, and more have demonstrated exceptional language understanding and generation capabilities. However, they are not immune to certain issues, one of which is hallucination. We will explore the causes behind hallucination in large language models, the potential risks it poses, and strategies to address these challenges.
Understanding Large Language Models
Before delving into the causes of hallucination, let's first establish what large language models are and provide some notable examples. Large language models are neural network-based architectures trained on massive datasets to understand and generate human language. They can be fine-tuned for various natural language processing tasks such as text completion, translation, question-answering, and even image generation.
Notable models like ChatGPT and Stable Diffusion, Google Bard, and Bing Chat have revolutionized the public eye to generative AI. These models have been trained on extensive corpora, encompassing billions of sentences, which allows them to capture intricate language patterns and generate contextually appropriate text. But they are not always 100% right.
What is Hallucination in Language Models
Hallucination refers to the phenomenon where language models generate outputs that are factually incorrect or deviate from the expected context. It is important to note that hallucination is not intentional but rather a side effect of the complex mechanisms underlying these models.
You may have seen social media posts of these large language models gaslighting the prompter on insisting that the AI’s response is incorrect. You may have also played with DALLE-2 or Stable Diffusion to see that the image generated misconstrued your prompt. DALLE-2 also cannot generate text on its images and will do its best to imagine text with symbols and globs.
There are a couple of reasons why this can happen in a language model even as big as GPT-3 or even the even larger GPT-4 (though less likely as you will learn).
Insufficient Training Data
One of the causes of hallucination in language models is the availability of limited or insufficient training data. Language models require a diverse and comprehensive dataset to learn accurate representations of language. When the training data is inadequate or lacks variety, the model may struggle to capture the nuances of language and generate accurate responses. This can result in the model filling in knowledge gaps with fabricated information, leading to hallucinations.
To address data limitations, researchers are exploring methods such as data augmentation, where synthetic data is generated to supplement the training set. Additionally, efforts are being made to curate high-quality and diverse datasets to improve the performance and reliability of language models.
Biases and Prejudices
Language models can also exhibit biases and prejudices, which can contribute to hallucinations. These biases stem from the biases present in the training data, which may reflect societal biases and prejudices. When the model lacks proper mitigation techniques, it may inadvertently generate content that aligns with these biases, even if they are factually incorrect or ethically problematic.
To tackle biases in language models, researchers and developers are actively working on techniques such as debiasing algorithms, which aim to reduce biased outputs. Ethical considerations and guidelines are being developed to ensure the responsible deployment of language models in various applications.
Contextual Ambiguity
Language understanding heavily relies on contextual information. However, accurately representing and interpreting context is a complex task for language models. Ambiguity in context can lead to hallucination, where the model generates responses that seem plausible in one interpretation but are incorrect or unrelated to the intended meaning.
The way large language models like ChatGPT work are similar to auto-complete. It uses various weights and statistics to predict the next best work depending on the factors such as prompt, previous tokens/conversations, and relevant data it is talking about. If you are ever in a chat with ChatGPT, you can continue the conversation with ambiguous questions, and it will do its best to answer depending on the topic previously discussed.
This can also affect how the model will react when previous prompts are swinging one way, and the intended or expected response get garbled by prior tokens.
Efforts are underway to improve context representation in language models by incorporating contextual embeddings and attention mechanisms. These techniques aim to enhance the model's ability to understand and generate contextually appropriate responses, reducing the occurrence of hallucinations.
Knowledge Gaps
Language models may encounter situations where they lack sufficient knowledge to generate accurate responses. In such cases, they may resort to fabricating information or making assumptions, leading to hallucinations. For example, if a language model lacks information about a particular event or concept, it may generate fictional details to fill the gaps.
Since the language model’s job is to predict the best words to say, it may be difficult for it to admit it doesn’t know versus spewing fabricated misinformation.
Strategies to address knowledge gaps involve leveraging external knowledge sources such as knowledge graphs, ontologies, and pre-existing databases. By integrating external knowledge into language models, their ability to provide accurate and informative responses as well as the capability to say it doesn’t know can be improved.
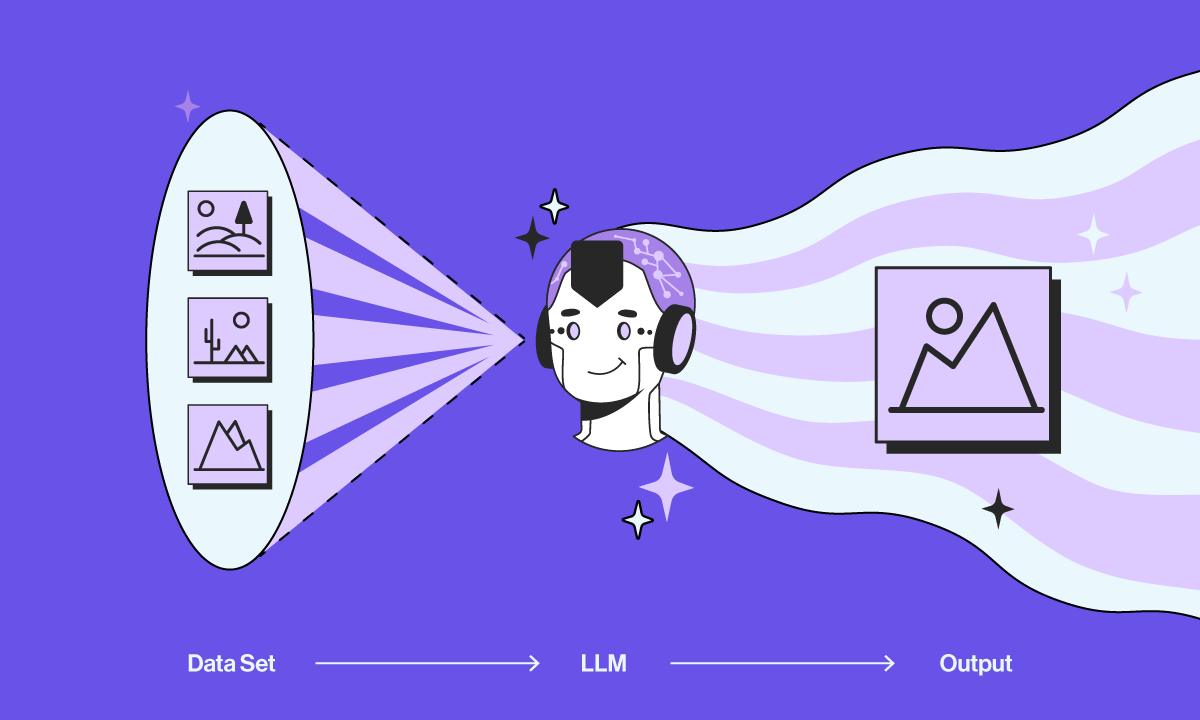
Adversarial Attacks
Language models are vulnerable to adversarial attacks, where malicious actors intentionally manipulate inputs to deceive the model and generate hallucinatory outputs. Adversarial attacks can exploit weaknesses in the model's architecture or training process, leading to unexpected and inaccurate responses.
You may have seen early attacks on newly released ChatGPT to explain how to perform an illegal task. ChatGPT would respond with warnings on not performing said illegal task and would not provide instructions. But once the prompter asks the model to pretend or assume a persona, it would have no problem providing instructions.
To mitigate the risks associated with adversarial attacks, researchers are developing robust defense mechanisms, including adversarial training and input perturbations. These techniques aim to enhance the model's resilience against adversarial manipulations and reduce the likelihood of hallucination. OpenAI’s ChatGPT has already patched this problem but should still be monitored closely to align with morality.
Balancing Creativity and Accuracy
Large language models are designed to generate creative and contextually appropriate text. However, there is a delicate balance between creativity and accuracy. In some cases, the pursuit of novelty and creativity can lead to hallucination, where the model generates imaginative but incorrect or nonsensical content.
To strike a balance, researchers are exploring techniques that encourage creative output while maintaining adherence to factual accuracy. Fine-tuning models and incorporating human-in-the-loop feedback can help guide the model's generation toward more accurate and reliable results.
However, if creativity is stifled, answers become less insightful, extremely robotic, and stoic. As a large language model designed to be a chatbot, conversations between ChatGPT, Bard, and Bing should flow naturally for it to be an effective tool for users.
Conclusion
Large language models have opened up new possibilities in natural language processing, but they are not immune to hallucination. The causes of hallucination in language models can range from neural network architecture complexities to data limitations, biases, contextual ambiguity, knowledge gaps, and vulnerabilities to adversarial attacks. Mitigating hallucination requires a multi-faceted approach, including diverse and high-quality training data, bias mitigation techniques, improved context representation, external knowledge integration, and robust defense mechanisms.
By addressing these challenges, we can ensure that large language models continue to provide accurate and contextually appropriate responses, reducing the risks associated with hallucination. As research and development in this field progress, it is crucial to consider the ethical implications and real-world impacts of language models to foster responsible and beneficial AI applications.
Impact on Real-World Applications
Hallucinations in language models can have significant consequences in real-world applications. In domains such as healthcare, finance, and legal, where accurate and reliable information is crucial, hallucination can lead to misleading or erroneous outputs. This can impact decision-making processes and potentially harm individuals or organizations relying on the language model's responses.
To mitigate the risks, careful validation and testing procedures are necessary before deploying language models in critical applications. Regular monitoring and feedback loops can help identify and address instances of hallucination, ensuring the reliability and trustworthiness of the model's outputs.
Future Developments and Research
The research community is actively working on advancing large language models and addressing the challenges associated with hallucination and increasing accuracy. Ongoing efforts include improving training methodologies, enhancing context understanding, reducing biases, and developing mechanisms to handle adversarial attacks. Additionally, collaborations between researchers, developers, and policymakers are vital to ensure the ethical and responsible use of language models.
As language models continue to evolve, it is essential to prioritize transparency, interpretability, and accountability. Striking a balance between innovation and addressing the risks associated with hallucination will pave the way for more reliable and trustworthy language models in the future.
The amount of data needed to train these large models are enormous with billions of parameters governing their foundation. Current models are delivering highly performant scores on academic and professional exams like the SAT, AP Tests, LSAT, GRE, and more. These models are the next technological advancement that changes the landscape of knowledge. Once models hallucinate, they lose credibility in their use. It is imperative that the future of these LLMs squash any trace of misinformation and unreliability to ensure a safe environment for the birth of Artificial General Intelligence.