What is a Generative AI
ChatGPT, DALLE-2, MidJourney, Stable Diffusion, and more are become extremely hot commodities due to their mind-boggling capabilities. Text-to-Image Generative AIs made a huge wave earlier this year displaying uncanny creativity to create original and coherent images. Now the AI chatbot ChatGPT pushes the limits of a personal assistant, exceeding the worldly capabilities of our smartphone assistants. We are in a technological revolution.
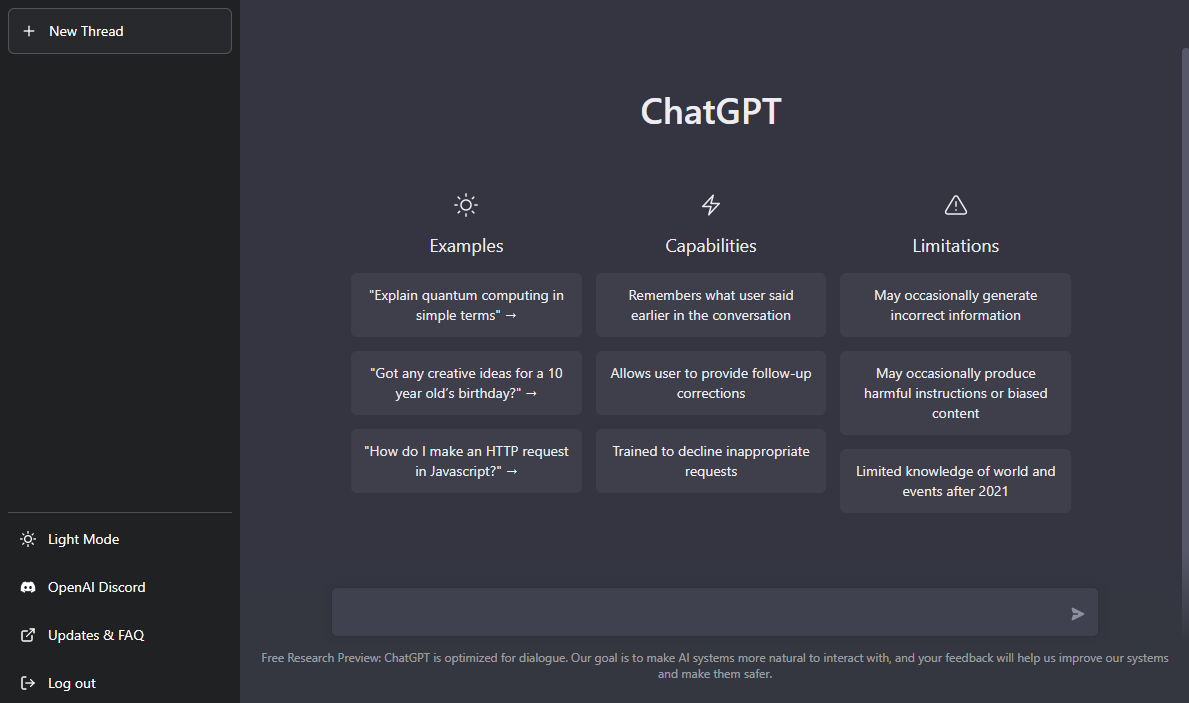
All these applications are built using an AI model called Generative AI. Generative AI has had a significant impact on many different fields and industries. And it is becoming more and more apparent with the OpenAI’s release of ChatGPT. With over 1 million users within a week, this was one of the fastest software applications adopted in recent history displaying the immense influence artificial intelligence has for the world moving forward.
Generative AIs in the Real World
But Generative AIs aren’t anything new. A generative AI is a type of artificial intelligence system capable of generating new data, such as images, text, or music, based on input data. These systems typically use deep learning algorithms, such as generative adversarial networks (GANs), to learn the underlying patterns in a given dataset and then generate new data that is similar to the input data.
There are websites that display a face of a person except the person doesn’t exist but is instead created by an AI. NVIDIA recently developed a frame generation technology for their graphics cards called DLSS 3.0 which is a type of generative AI that extrapolates frames and creates very accurate guesses to fill in low FPS gameplay.
We have also used Generative AI to remaster old movies and TV shows with better image quality or increased the framerate of animation for smoother more cohesive playback. Generative AI isn’t anything new but OpenAI has been brought to light so that the average person can interact with one with DALLE-2 and ChatGPT.
Some of the ways it has changed the world include:
- Generative models have been used to create new forms of art, such as music and visual art, by generating original content based on a set of input data.
- In healthcare, generative AI has been used to develop new drugs and discover new insights into diseases.
- Generative AI has also been used in the field of finance to develop new investment strategies and automate trading processes.
- In transportation, generative AI has been used to develop self-driving cars and other forms of autonomous vehicles.
- Generative AI has also been used in many other fields, such as natural language processing and computer vision, to improve the performance of these systems and develop new applications.
Impact of Generative AI
Overall, generative AI has had a profound impact on many different industries and has opened up new possibilities for research and innovation. ChatGPT is generalized to help users code, search, and offer near artificial general intelligence. DALLE-2 offers creativity inspiration for artists and creators with AI-generated images from text prompts. There are also many new companies looking to develop and create AIs to improve our workflow.
Generative AIs in healthcare can assist scientists with unbiased diagnoses based on client symptoms and medical history. In manufacturing, AIs can be utilized to assist with design optimization, material efficiency, and production speeds. It is also used in the finance industry to automatically detect fraudulent activity by analyzing purchase habits.
All these use cases help the productivity of our work by reducing errors, reducing bias, providing consistency, and enabling humans to tackle more complex tasks. There is a very large and addressable market for developing and training a generative AI model.
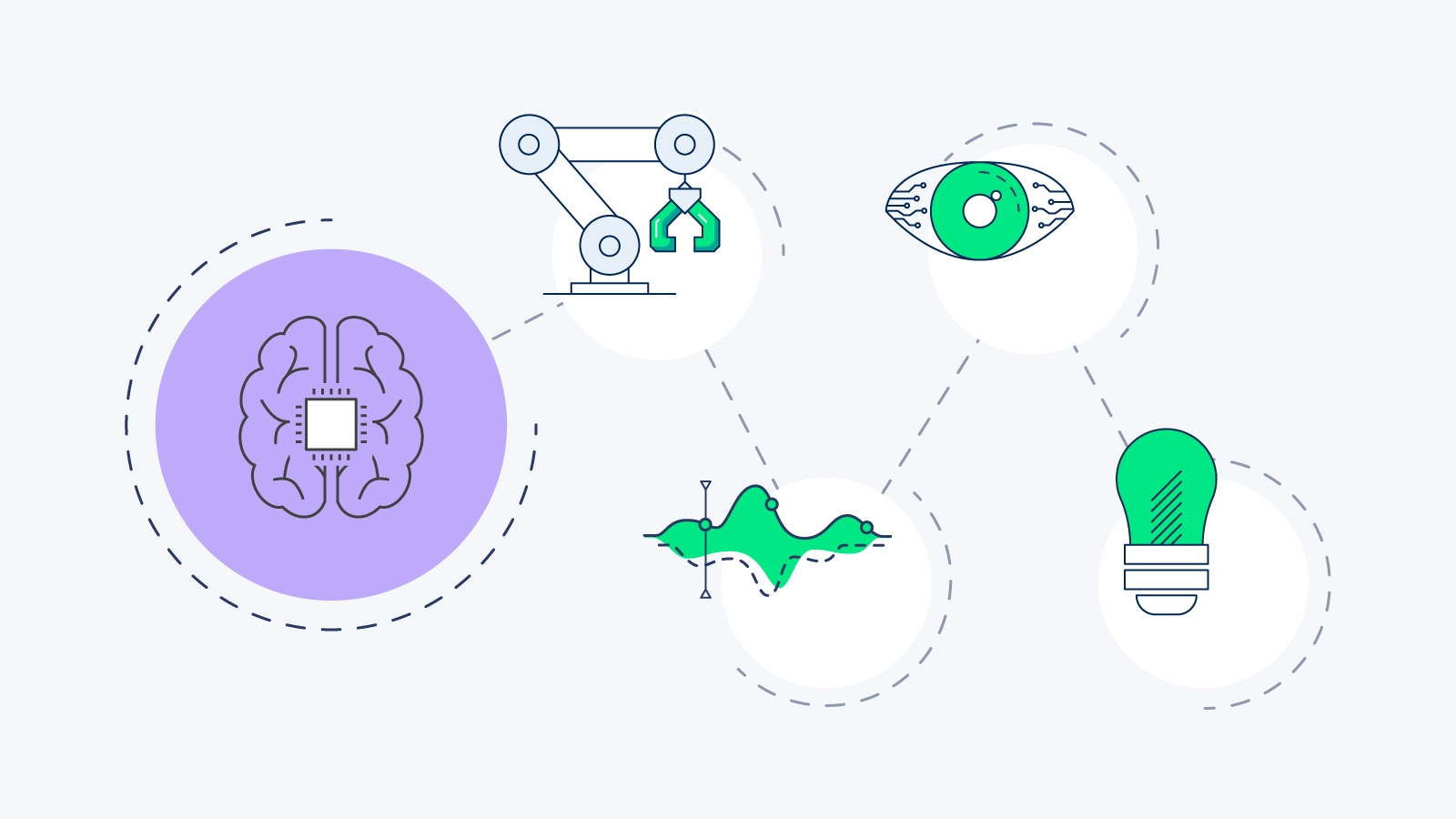
Getting Ready to Train a Generative AI
Training a generative AI model typically involves providing the model with a large amount of data to learn from. The model is then trained using a variety of techniques, such as unsupervised learning or reinforcement learning, to learn the underlying patterns and structures in the data.
This process can be computationally intensive and may require the use of specialized hardware and GPUs to train the model efficiently. Once the generative model has been trained, it can generate new data that is similar to the training data for accurate outputs or further training.
There are several effective methods for training a generative AI model, and the choice of method will depend on the specific goals of your project and the characteristics of the data. A few commonly used approaches include:
- Generative adversarial networks (GANs): GANs are a popular method for training generative models. They consist of a generator network and a discriminator network, which are two neural networks trained to work together to generate new samples that are similar to the real ones. The generator network generates new samples similar to the data set while the discriminator network distinguishes which samples are generated and which ones are real. As the GAN continues to train the generated samples will begin to be very similar to the sample.
- Variational autoencoders (VAEs): VAEs are another widely used method for training generative models. They learn a low-dimensional representation of the data to generate new samples by sampling from a simple distribution (such as a standard normal distribution) and then transforming the samples using the learned representation.
- Normalizing flows: Normalizing flows are a type of generative model that use invertible transformations to map data points from a simple distribution to a more complex one. They are particularly useful for generating high-dimensional data and can produce samples that are highly realistic and diverse.
- Autoregressive models: Autoregressive models are a class of generative models that generate new data by predicting the next value in a sequence based on the values that come before it. They are often used to generate time series data and can be trained using techniques such as gradient descent and backpropagation.
In addition to these methods, it is important to carefully preprocess and prepare your data before training a generative model. This can include tasks such as normalizing the data, removing outliers, and splitting the data into training and test sets.
If you are interested in the field of deep learning and training your very own generative AI, SabrePC offers developer workstations or up to 10 GPU server nodes for scale-up and scale-out operations for effective training and deployment. Contact Us to start your Deep Learning Journey today!